AI: Because Weathermen (and Brand Managers) Can’t Read a Cloud
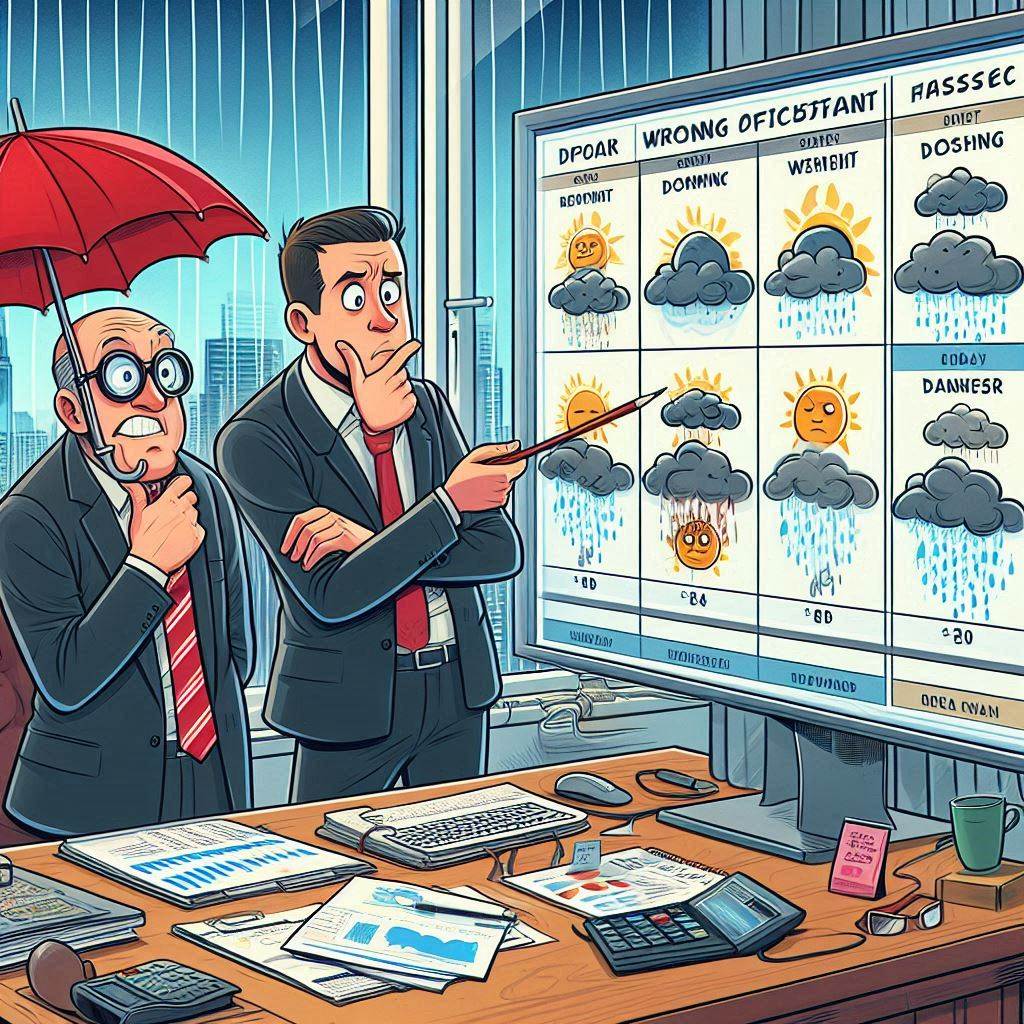
Preamble
The IMD and SKYMET had a field day predicting heavy, torrential rains for Ahmedabad during June, July, and August 2024. They did this about four or five times, and each time, the Sun God seemed to be having a chuckle at our expense. The city was bathed in bright sunshine, and the only downpour I witnessed was the sweat trickling down my forehead from the scorching heat and humid weather.
It reminded me of my less-than-stellar days in brand planning, when my predictions were about as accurate as a weather forecast in the Bermuda Triangle.
I even joked with my wife, Veena that I might have a future as a weatherman, given how consistently wrong my forecasts in brand plans were. Those nostalgic memories have inspired me to write this essay, a testament to my uncanny ability to predict the opposite of what actually happens.
Those nostalgic moments are now prompting me to write this essay.
Weathering the Storm of Predictions
In the sweltering summer of 2024, two friends found themselves in a peculiar predicament. Raj was a weatherman and Priya was a brand manager in Canopus Pharma Ltd.
Raj, the eternal optimist of the Indian Meteorological Department, stood before his weather maps, scratching his head. His confident predictions of a bountiful week of very heavy to heavy echoed by the folks at SKYMET had evaporated like morning dew.
El Niña, it seemed, had played a cruel joke, leaving the Ahmedabadi’s staring at cloudless skies and River Sabarmati being fed with waters from the Narmada.
Meanwhile, in a sleek Ahmedabad office of Canopus Pharma, Priya gazed forlornly at her sales projections. Like Raj’s rain gauges, her charts stubbornly refused to align with reality. Her carefully crafted brand plan, once a beacon of hope, now seemed as reliable as a weathervane in during the monsoon storms.
As Raj’s rain dance proved futile and Priya’s sales graph resembled a disappointing ECG, both realized a harsh truth: in the world of predictions, humans often fall short. But what if there was a way to clear the foggy crystal ball?
Enter generative AI with a knack for number-crunching and pattern recognition. While it couldn’t make the rain fall or pills fly off shelves, it offered a new approach to forecasting.
For Priya, leveraging generative AI meant diving deep into a sea of data. Historical sales trends, market dynamics, competitor activities, and even social media sentiments – generative AI could analyze it all in the blink of an eye.
Unlike human bias that might focus on recent wins or losses, generative AI objectively weighed every factor. But generative AI’s real magic lay in its ability to spot correlations humans might miss. Perhaps flu season trends could predict flu and cold medicine sales, or social media buzz could indicate emerging health concerns. By connecting these dots, generative AI could paint a more nuanced picture of the future.
Moreover, generative AI could run countless simulations, testing various scenarios and their impact on brand performance.
What if a competitor launched early? What if a new study boosted product credibility? Generative AI could model these situations, helping Priya prepare for multiple futures.
As Priya embraced this AI-augmented approach, her forecasts began to align more closely with reality. However, she soon discovered an important caveat: generative AI’s predictions, like those of all AI systems, were fundamentally based on historical data. In the ever-changing pharmaceutical landscape, past performance didn’t always guarantee future results.
Priya realized that while generative AI could process vast amounts of information and identify patterns humans might miss, it couldn’t account for unprecedented events or sudden market shifts. A breakthrough drug from a competitor, an unexpected regulatory change, or a global health crisis could upend even the most sophisticated AI-generated forecast.
This revelation led Priya to develop a more nuanced approach. She learned to blend generative AI’s data-driven insights with her own industry expertise and intuition.
Generative AI became a powerful tool in her arsenal, but not a crystal ball. It provided a solid foundation for predictions, which Priya then refined based on her understanding of current trends, emerging technologies, and the human factors that drive the pharmaceutical market.
Back at the weather office, Raj nodded in understanding when Priya shared her learning’s. He too knew that while advanced models could crunch numbers and analyze patterns, the chaotic nature of weather – much like the pharmaceutical market – meant that some degree of uncertainty would always remain.
In the end, whether predicting rain or sales, the forecast called for a 100% chance of generative AI assistance – with a healthy dose of human wisdom to interpret the results. As Priya and Raj discovered, the most accurate predictions come not from generative AI alone, but from a thoughtful combination of artificial intelligence and human insight, especially when navigating uncharted waters.